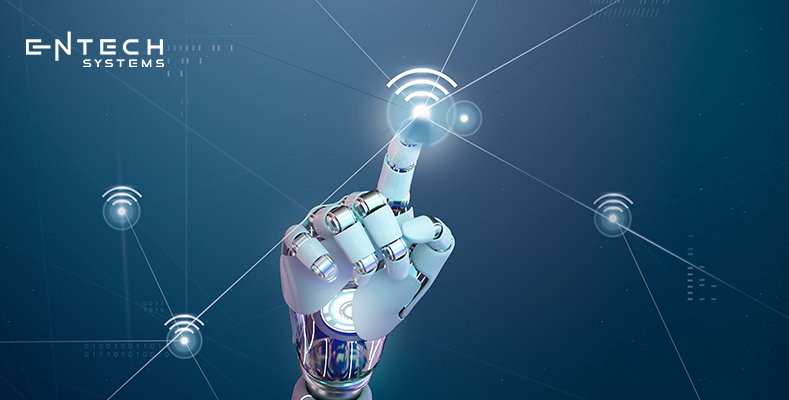
Exploring the Next Wave of AI-Driven Network
Exploring the Next Wave of AI-Driven Network Management Tools
Networks have become increasingly intricate, spanning from cloud-based architectures to hybrid systems. The conventional paradigms of network management need to catch up. Tools for network management based on advanced artificial intelligence have become an effective answer to this issue.
These include optimising performance, improving security, and alleviating workload on IT staff. This blog looks into the forthcoming wave of tools powered by artificial intelligence and their capabilities to transform practices.
The Evolution of Network Management
The period for assessing what network management is all about has gone. Before, network management was predominantly centralised.
It meant that system administrators were active in every element of the systems. They monitored systems, they troubleshoot issues, or they uploaded patches or configs.
The growth of the organisation system contributed to the strain of network infrastructure which could no longer be controlled manually.
Network management utilities to simplify the deployments with smart data analysis-based actions. More to the point, these utilities are built for moving systems where the population of devices and data constantly increases.
Key Features of AI-Driven Network Management Tools
The upcoming generation of AI-enabled solutions offers several aspects that can be seen as extending conventional network management systems.
Predictive Modeling
AI is capable of sifting through past performances and trends of the systems to know and anticipate what the future holds.
For instance, in case there is a server that registers high traffic volumes, especially at the peak periods of the day. AI tools can detect this trend and even recommend that more resources be added in advance.
This ability proves to be very useful in keeping the business operational. As it curbs the rate at which the users experience segregation and the transition is seamless.
Self-Organising Networks
AI-based systems can automatically govern all the parameters for the network tuning, following a certain performance specification, and/or requirements.
Due to this, there is no room for configuration-induced errors caused by people. One of the factors to the new network and optimised configurations given the varying demands.
Anomaly Detection and Response
One of the most critical benefits of AI in network management is anomaly detection. AI can monitor traffic and usage of the network over time and learn what is normal. And detect any irregular behaviours that could pose a danger, such as a breach or failure within the system. These systems can then warn the IT department, or solve the problem by themselves, to avoid any losses or breaches.
Smart Load Balancing
With the development of workload distribution systems, it is becoming more difficult to maintain the proper allocation of resources.
Intelligent balancing of load using AI tools helps to optimise network traffic distribution to avoid congesting and overloading of the servers. This consequently enhances performance and reduces the latency experienced by end users.
Self-Recovery Networks
The following generation of network management tools has been developed with self-healing functions. AI is capable of identifying and addressing basic network problems without people’s help. This can be restarting an active service that has stalled or diverting any traffic away from a faulty server. These AI tools can troubleshoot issues that would otherwise cause a halt in services ensuring that networks run round.
AI-Powered Security Enhancements
The upsurge in cyber threats to organisations has made network security a foremost concern. Today’s AI network management systems come with comprehensive security features that extend beyond firewalls.
Threat Detection and Prevention
By leveraging AI algorithms, it is possible to scan millions of terabytes effectively without missing a drop. So real-time information can capture, trends that show how viruses or harm are one step away discerned.
In contrast to conventional security systems that work through ‘if-then’ logic gates, artificial intelligence weapons systems ABM ‘intelligent’ defence may change the rules forever as, even outside a pre-defined threat classification, attacks can assign ‘degrees of abnormality’ relative to normal network patterns of behaviour.
Automated Incident Response
Once any evidence of a security compromise is identified, tools powered by artificial intelligence will often have the capacity to activate a pre-emptive mitigation strategy.
This may involve disconnecting affected endpoints and black-holing certain ranges of IP addresses. Such automated countermeasures add the benefit of lessening the time between identification and suppression.
Compliance Monitoring
Without the need for human intervention, AI systems can help to keep the entire network in line. The relevant standards and policies by periodically checking tactical measures such as system configurations and access restrictions.
If the system detects a breach in compliance, it may correct the breach automatically or notify the administrators to do so.
The Role of Machine Learning in Continuous Optimisation
A prominent characteristic of most AI-powered network management applications is that they can develop over time. Machine learning programs constantly monitor the network, looking for inefficiencies or places where performance is otherwise constrained. At this point, these systems would simply change settings, routing protocols and/or methods of load distribution to improve performance levels on their own.
- This constant ‘tuning’ feature is important because it helps overcome the limitations of static networking, which cannot evolve fast enough to serve the changing business requirements. As the network grows to include new devices, new users, and new applications, the AI tools can change dynamically to ensure that they are properly tuned for performance across the board.
- Nevertheless, the promise of AI-powered tools for the management of the network is clear, but their actual adoption presents difficulties.
- For any sort of AI systems to work, they load with heavy amounts of data. There is a need to ensure that such data is not only secured but also does not violate any privacy rights.
- This states the difficulty faced by conversion in that most companies have a portion of their network infrastructure held within legacy systems. AI-driven applications against these conventional systems do not have a significant integration challenge.
- The cost of the AI-powered solutions concerns not just the software, which many expect but also almost all trained personnel to collect and analyse the AI outputs.
Nevertheless, the benefits in the maintenance costs such as less revenue loss due to downtimes. Low costs of operations are often far higher than these early hitches.
Conclusion
Tools driven by artificial intelligence in managing networks herald the future of management, which is cost-effective, secure and scalable. In such economies, predictive jobs, anomaly Olympics and self-healing networks among others radically. Alter how businesses manage their digital assets.
Nevertheless, these issues still exist, and the potential of AI to improve networks, cut costs and enhance security. The impact of AI on the sphere of network management will continue to change. It is such developments that will lead to placing new limitations on the existing IT infrastructure management.